New, new, new [ June / September 2019 ]
- A story of ATLAS computing in the ATLAS25 book ! [pages 213-223]
- The ATLAS Run 2 trigger workflow summarised in an EPS prize winning poster ! [ photos ]
- Open days ATLAS computing corner: posters on data processing and computing infrastructurecomputing
- Open days CERN data centre information sheet
2018: design, facts and figures
ATLAS reports once per year to the LHC Computing Resource Scrutiny Group (CRSG) and the informations given in these reports can be considered as scrutinised and approved/public: check the chapter Interactions with the CRSG in the Computing Model Twiki. 2 files are attached below: a "reader's digest"of the April 2018 report to CRSG, updates of the exising brochures.
- CPU: a typical laptop has 2 cores, a medium size T2 center such as the LPNHE Grif has 3500 cores. Each core provides ~ 10 HS06. ATLAS 2017 resources ~ 2.8 M HS06 ( 420 k @CERN, 820 k @T1, 1500 k @T2 ) ... i.e. 140 k laptops.
- Storage: the 2017 raw data volume is 10 Petabytes ( recording rate is driven by time to write on tape, ~ 1 kHz and 2 GB/s). But we also have derived collision and simulation data --> Data volume on disk ~ 170 Petabytes, on tapes ~ 160 PetaBytes. Given that resources are always used to their maximum, an advanced monitoring of data usage patterns and formats by scientists was implemented. Raw data are kept for ever, but derived data have a lifetime.
- Distributed computing: ~ 250 k tasks are running in parallel (with automatic submission, monitoring & problem detection, including by Machine Learning). Per week: T0 export ~ 1 PB; data movements for production and analysis ~ 5 PB ; user analysis ~ 10 to 15 PB.
--> See below the attached "computing brochure updates"
2018: run 2 achievements
Ressources growth: the CERN data center in 2006 = 5000 PC and 5 PB storage. In 2012 CERN had 65k core CPU, representing 20% of the total LHC computing capacity --> huge effort from the whole community to catch up with the LHC stellar performance (twice design luminosity) and higher pileup than expected (up to ~60). Some of it is described the CERN courrier [2016], fresh numbers and facts are updated monthly on the IT web site (top right box "key infos and numbers")
Change of work model for the grid: thanks to the amazing network capacity increase, the Run 1 model (which defined precise T0-T1-T2 hierarchy & roles) was replaced for Run 2 by a flexible "resources driven" model of ~ 30 "Nucleus + Satellites" where tasks go where they fit best. The story will be told in the chapter 7 of the ATLAS book (pages 14 to 20).
Integration of non standard resources such as HPC's and Commercial Cloud is already a reality. In 2017, 15% of the ATLAS simulation was done on the cloud, 10% on HPC. Recent examples were presented in conferences
- Partnership with Google: see the first few slides of this talk , introducing ATLAS @ the Google conference Next'2018
- Running ATLAS simulation on HPCs: non technical July 2018 overview talk
Keeping up with simulation needs: high quality run 2 analyses on the full LHC physics spectrum... but also support for 6 TDR of upgrade phase II . In 2017, event generation was 15% of the total CPU, simulation was 45%, reconstruction of simulated events 12% --> total: 72%
2018: future challenges
- The computing landscape has changed... need to rethink the mix between "home made" software and new / ultra specialised open source & commercial sector tools. Difficulty: our software and computing combine data of many different sources, formats and lifetime ( event data, calibration constants, output data quality histograms, run and files metadata bookkeeping).
- LHC High lumi: the roadmap discussed with the whole community ( Hep Software Foundation ) is described in a recent article in the CERN Courrier
"Big data and extreme computing", the HPC report quoted in the last slide of the latest ICHEP plenary talk on Sw & Computing (July 2018, slide 11), discusses the differences between our data-driven computing model, other scientific disciplines and the commercial sector. A few points are listed in this readers digest.
Stories, images and videos
On public web sites:
- Check ATLAS computing related news
- How Grid computing helped to find the Higgs: science node article 2012 (ex iSGTW - International Science GRid This Week)
- The LHC's worldwide computing: CERN courier 2013
- SKA and CERN cooperate on extreme computing: CERN courier 2017
- Time to adapt to big data: CERN courier 2018
"Explaining our computing to the public": a BoF session @ CHEP 2018
An informal discussion took place at CHEP 2018, using the BoF ("Birds of Feathers") format. A follow up working group should be put in place for 2019, but meanwhile the following points were made:
- Young people think that they have "everything" in their smartphones and have no idea (and very little curiosity) on what happens behind the GAFA magic: showing them techical aspects & objects such as fibres (transmit the data), disks and tapes (store the data) is engaging. This is the strategy followed in the CERN data center visit point, and every visit raises a - lot - of questions on comparisons with everyday life.
- HEP has always tuned its computing to the existing technologies, but pushed beyond their industrial specs: vintage pictures, web invention, grid as "cloud before the cloud" illustrate this very well. For the future, if HPC and the cloud are the ways to go... we will adapt and push them to their best, because this is what we always do.
- Holding & accessing data: our data are very valuable, because running the collider and experiments cost. We need to access them fast (conferences) but also over a long time (reprocessing, filtering over larger and larger satistics). The problem of data preservation and replication (against loss), as well as safe identification (against malicious hacks) and content delivery network (stability) are thus crucial.
- Analysis is about filtering, compactification, investigation... and "making sense out of all these numbers". Our data are not a flat list of images or text, and analysis is not a set of relational querries. Data analysis means digging into details in a flexible way, and transforming the information at each processing step to encapsulate the understanting (calibration, alignment, signal processing) as simply as possible... and thus save resources (cpu, storage, humain brain time). A lot of the developper's work and creativity goes into the "filtering" software development and each tool performance improvements.
- Getting the right comparisons ? Piling CD-Rom up to the moon may not make much sense any more, but illustrations of what Mega and Peta mean still do and the most commonly used is the human hair diameter ~ 100 microns in average --> 1 k = 10 cm; 1 M = 100 m; 1 T = 100 km; 1 P = 100 000 km = 1/3 of the Earth-Moon distance.
- A video playlist was setup for the CHEP 2018 outreach event, it is attached below in case someone finds it useful.
Latest update of this page: September 2018.
The first slide below is taken from the latest ICHEP plenary talk on Sw & Computing (July 2018, slide 11). The second is taken from CERN brochures.
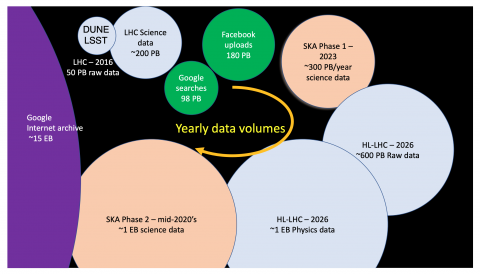
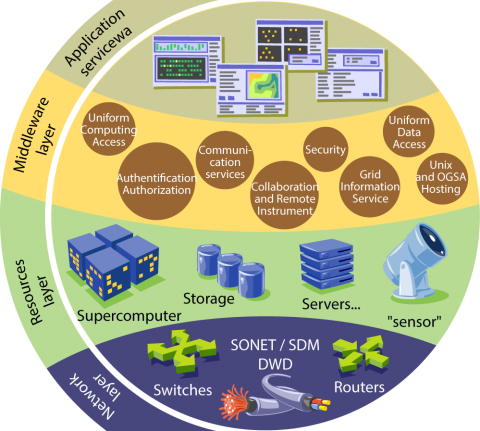